AI-Fakes Detection Is Failing Voters in the Global South
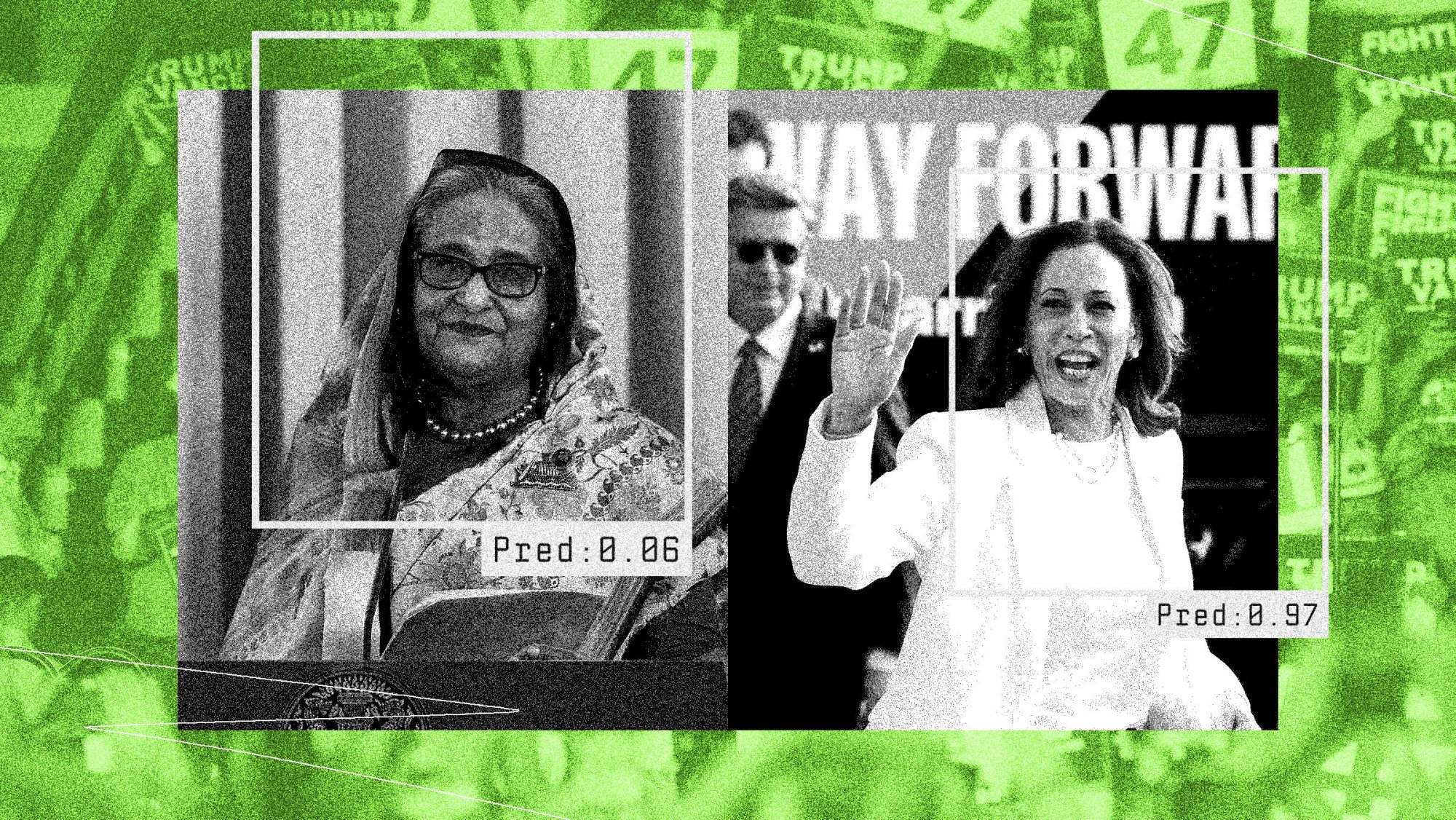
Politics tamfitronics
Recently, former president and convicted felon Donald Trump posted a series of photos that appeared to show fans of pop star Taylor Swift supporting his bid for the US presidency. The pictures looked AI-generated, and WIRED was able to confirm they probably were by running them through the nonprofit True Media’s detection tool to confirm that they showed “substantial evidence of manipulation.”
Things aren’t always that easy. The use of generative AI, including for political purposes, has become increasingly common, and WIRED has been tracking its use in elections around the world. But in much of the world outside the US and parts of Europe, detecting AI-generated content is difficult because of biases in the training of systems, leaving journalists and researchers with few resources to address the deluge of disinformation headed their way.
Detecting media generated or manipulated using AI is still a burgeoning field, a response to the sudden explosion of generative AI companies. (AI startups pulled in over $21 billion in investment in 2023 alone.) “There’s a lot more easily accessible tools and tech available that actually allows someone to create synthetic media than the ones that are available to actually detect it,” says Sabhanaz Rashid Diya, founder of the Tech Global Institute, a think tank focused on tech policy in the Global South.
Most tools currently on the market can only offer between an 85 and 90 percent confidence rate when it comes to determining whether something was made with AI, according to Sam Gregory, program director of the nonprofit Witness, which helps people use technology to support human rights. But when dealing with content from someplace like Bangladesh or Senegal, where subjects aren’t white or they aren’t speaking English, that confidence level plummets. “As tools were developed, they were prioritized for particular markets,” says Gregory. In the data used to train the models, “they prioritized English language—US-accented English—or faces predominant in the Western world.”
This means that AI models were mostly trained on data from and for Western markets, and therefore can’t really recognize anything that falls outside of those parameters. In some cases that’s because companies were training models using the data that was most easily available on the internet, where English is by far the dominant language. “Most of our data, actually, from [Africa] is in hard copy,” says Richard Ngamita, founder of Thraets, a nonprofit civic tech organization focused on digital threats in Africa and other parts of the Global South. This means that unless that data is digitized, AI models can’t be trained on it.
Without the vast amounts of data needed to train AI models well enough to accurately detect AI-generated or AI-manipulated content, models will often return false positives, flagging real content as AI generated, or false negatives, identifying AI-generated content as real. “If you use any of the off the shelf tools that are for detecting AI-generated text, they tend to detect English that’s written by non-native English speakers, and assume that non-native English speaker writing is actually AI,” says Diya. “There’s a lot of false positives because they weren’t trained on certain data.”
But it’s not just that models can’t recognize accents, languages, syntax, or faces less common in Western countries. “A lot of the initial deepfake detection tools were trained on high quality media,” says Gregory. But in much of the world, including Africa, cheap Chinese smartphone brands that offer stripped-down features dominate the market. The photos and videos that these phones are able to produce are much lower quality, further confusing detection models, says Ngamita.
Gregory says that some models are so sensitive that even background noise in a piece of audio, or compressing a video for social media, can result in a false positive or negative. “But those are exactly the circumstances you encounter in the real world, rough and tumble detection,” he says. The free, public-facing tools that most journalists, fact checkers, and civil society members are likely to have access to are also “the ones that are extremely inaccurate, in terms of dealing both with the inequity of who is represented in the training data and of the challenges of dealing with this lower quality material.”
Generative AI is not the only way to create manipulated media. So-called cheapfakes, or media manipulated by adding misleading labels or simply slowing down or editing audio and video, are also very common in the Global South, but can be mistakenly flagged as AI-manipulated by faulty models or untrained researchers.
Diya worries that groups using tools that are more likely to flag content from outside the US and Europe as AI generated could have serious repercussions on a policy level, encouraging legislators to crack down on imaginary problems. “There’s a huge risk in terms of inflating those kinds of numbers,” she says. And developing new tools is hardly a matter of pressing a button.
Just like every other form of AI, building, testing, and running a detection model requires access to energy and data centers that are simply not available in much of the world. “If you talk about AI and local solutions here, it’s almost impossible without the compute side of things for us to even run any of our models that we are thinking about coming up with,” says Ngamita, who is based in Ghana. Without local alternatives, researchers like Ngamita are left with few options: pay for access to an off the shelf tool like the one offered by Reality Defender, the costs of which can be prohibitive; use inaccurate free tools; or try to get access through an academic institution.
For now, Ngamita says that his team has had to partner with a European university where they can send pieces of content for verification. Ngamita’s team has been compiling a dataset of possible deepfake instances from across the continent, which he says is valuable for academics and researchers who are trying to diversify their models’ datasets.
But sending data to someone else also has its drawbacks. “The lag time is quite significant,” says Diya. “It takes at least a few weeks by the time someone can confidently say that this is AI generated, and by that time, that content, the damage has already been done.”
Gregory says that Witness, which runs its own rapid response detection program, receives a “huge number” of cases. “It’s already challenging to handle those in the time frame that frontline journalists need, and at the volume they’re starting to encounter,” he says.
But Diya says that focusing so much on detection might divert funding and support away from organizations and institutions that make for a more resilient information ecosystem overall. Instead, she says, funding needs to go towards news outlets and civil society organizations that can engender a sense of public trust. “I don’t think that’s where the money is going,” she says. “I think it is going more into detection.”
Discover more from Tamfis Nigeria Lmited
Subscribe to get the latest posts sent to your email.