NASA Space Technology
As a machine learningscientist at NASA, Hamed Valizadegan once trained an algorithm to examine images of blood vessels in astronauts’ retinas, improving efforts to understand vision changes in microgravity. It was important work, but Valizadegan, who never lost his childhood love of the night sky, couldn’t shake his desire to study the stars.
“I could watch the sky for hours, contemplating the meaning of life and whether we are alone in this vast universe,” he says. Early on, however, his space scientist colleagues seemed reluctant to embrace artificial intelligence as a tool for exploring the cosmos. That may be because advanced algorithms don’t typically show their work. Sophisticated AI systems are inspired by the brain, so individual synthetic “neurons” make computations and then pass information to other nodes in the network. The resulting systems are so dense with calculations it can be impossible to know how they arrive at answers. That black box quality, Valizadegan says, was a turnoff to scientists who embraced historical standards for ultraprecise modeling and simulations.
But modern astronomy was reaching a bottleneck. Telescopes in space and on Earth collect so much information that humans can’t decipher it quickly, or even at all. And future observatories were being planned that would only flood the field with more observations. Take the Vera C. Rubin Observatory in Chile, which scientists first proposed building in 2001. Starting in 2025, it will image the whole sky every three nights with the world’s largest camera, with a resolution of 3,200 megapixels. It’s expected to capture data on one million supernovae every year, as well as tens of thousands of asteroids and other celestial objects. How could any number of human scientists possibly study them all on their own?
(How the space race launched an era of exploration beyond Earth.)
In 2014 Valizadegan teamed up with astronomer Jon Jenkins, who invited him to join a more automated search for another Earthlike planet in our galaxy. It was just the type of dreamy project Valizadegan was hoping for.
While life might exist in strange forms on planets unlike our own, scientists have set their sights on finding the familiar: a rocky world orbiting a star, with a stable atmosphere and liquid water. But discovering such a planet is literally an astronomical problem. Some estimates put the number of planets in the Milky Way in the hundreds of billions—with only some small but unknown proportion of them being Earthlike.
On this quest, humanity is off to a relatively slow start. Astronomers found the first planet orbiting a star other than our own—an exoplanet—in 1995. Efforts accelerated during the 2010s with the Kepler Space Telescope, which peered at 150,000 stars in one small patch of sky for nine years, rotating occasionally to scan a new section of space. Its successor, the Transiting Exoplanet Survey Satellite, was launched into space in 2018 to observe much more of the sky, focusing on about 200,000 stars closer to Earth.
(In the hunt for alien life, this planet just became a top suspect.)
Even with these space-based observatories, verifying that a planet orbits another star is time-consuming and difficult. These telescopes can’t visualize the planet itself—just as how any common telescope can see Jupiter or Saturn. Instead, they confirm its existence indirectly. That involves measuring almost imperceptible blips in a star’s brightness that could indicate a passing planet. Astronomers scrutinize changes in starlight, known as light curves, to identify potential planets. Then, to prove one exists, Earth-based telescopes measure how a star wobbles under its planet’s gravitational tug. Once a planet is found, understanding what it is like is even trickier. But astronomers can make assumptions based on size and distance from stars.
Thanks to these painstaking efforts, astronomers now know of at least 5,600 planets orbiting distant stars in the Milky Way. Some are huge gas giants bigger than Jupiter and Saturn; some are hot rocks smaller than Mars; most are worlds made of gas, rock, or both, often between the sizes of Earth and Neptune.
None resemble home. None have the conditions or the chemicals necessary for life as we know it. But AI could reveal something different, because it can take an even deeper look.
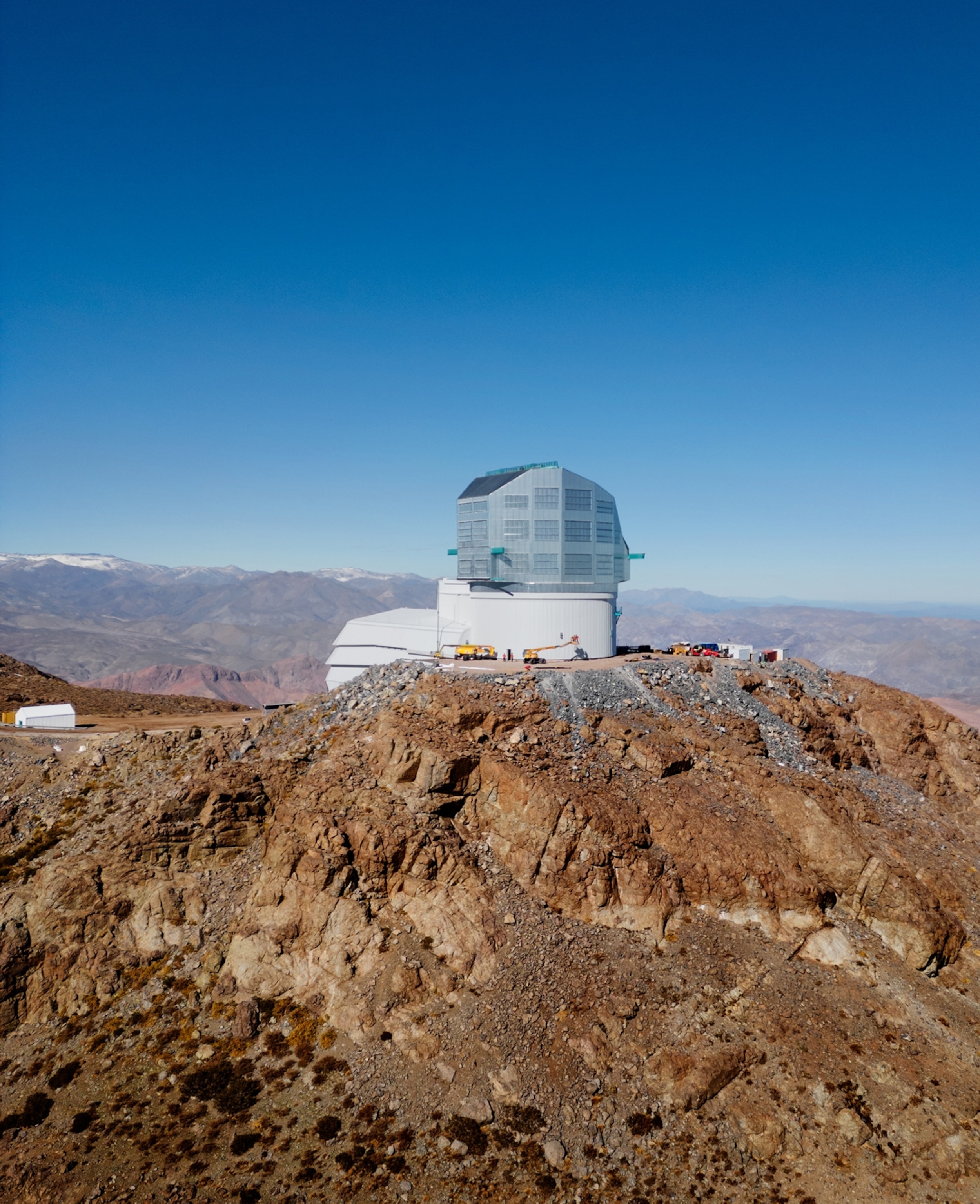
Data Deluge
Photograph by Spencer Lowell
In 2018 Valizadeganand his team began building a machine learning program to speed up exoplanet-hunting efforts. They trained the software on data signifying confirmed planets as well as false positives, like binary stars that eclipse each other and can be mistaken for passing planets. They called it ExoMiner and put it to the test on the Kepler telescope’s archive of observations.
“I did not know how much we were going to be rewarded,” Valizadegan says. But his model quickly identified 370 previously unknown exoplanets. “At first there was a lot of resistance from the [exoplanet] scientists, who said, ‘No, this should not be labeled as a planet.’ But over time, they got more confident.” ExoMiner has yet to be overruled, he says.
None of these 370 new planets are like Earth—or any other planet in our solar system, for that matter. One new world, dubbed Kepler-495 c, is about twice the size of Earth and zips around its sunlike star at a blistering pace, every six days. Another, called Kepler-27 d, is almost as big as Neptune, or about eight times as big as Earth, and experiences a speedy six-and-a-half-day year. Fried by their stars’ heat and radiation, these planets are likely uninhabitable.
Valizadegan says ExoMiner is just the start of using AI to solve this ultimate needle-in-a-haystack problem. A new generation of planet-hunting telescopes launching in the next decade will bring ever vaster quantities of starlight down to Earth. Future AI planet spotters, building on ExoMiner’s success, are also in development. In fact, researchers now believe that AI can be useful in hunting for not merely new worlds but also conditions most likely to host life.
In 2020 Lisa Kaltenegger,an exoplanet astrophysicist and director of the Carl Sagan Institute at Cornell University, and collaborator Dang Pham wondered if machine learning systems could be trained to pinpoint life-enabling resources like water—something ExoMiner cannot do. “If you find ice, you can infer water,” Kaltenegger says. “If you can find clouds, you infer water. So we asked, how good is it in finding water, clouds, and ice?”
Kaltenegger and Pham used measurements of the Earth’s atmosphere to simulate exoplanets with a rocky surface, water, clouds, and ice. They also trained an algorithm to look for a sign of life called a red edge, wavelengths of light that plants reflect back into space.
They found their software could detect the existence of life in a simulated atmosphere about three-quarters of the time, which could greatly improve the initial hunt for another Earth. “I thought it would be very, very hard to do, but machine learning algorithms are quite effective in finding patterns in the data,” Kaltenegger says. (The computer programs were best at spotting the telltale signs of leafy plants and less reliable when looking for evidence of lichen, tree bark, or biofilm.)
There are caveats. These algorithms cannot provide absolute certainty. Rather, one could estimate that some percentage of a planet’s surface is covered with life. That’s not the same as a discovery, Kaltenegger points out. Instead, it’s a helpful clue.
NASA Space Technology You May Also Like
“It’s not going to be like, AI said we found an Earthlike planet,” she explains. “AI is going to bring it to the level where some real people are going to have to look at it.” Human scientists will still need to point more telescopes toward the planet and look for chemical signatures that could indicate life is there.
Ultimately, real people will be the ones deciding what such a discovery means.
(Europa is an icy ocean world—and NASA is finally going to explore it.)
Valizadegan’s work is justone stunning example of how AI is giving us a more detailed view of the cosmos. Only a few years ago, scientists from an international team called the Event Horizon Telescope published the first image of a black hole. The effort involved hundreds of researchers stitching together data from radio telescopes placed around the world. The resulting image, however monumental, appeared blurry because of the telescopes’ limitations.
A computational astrophysicist and a member of that team, Lia Medeiros built an algorithm that finds patterns in the radio data and creates a new version of the image. The algorithm, called PRIMO, did not sharpen the image in the way a photographer might eliminate blurriness using Photoshop. Rather, it put together a brand-new image, as if a Photoshop user built a fresh picture. The result is a higher definition image than the one produced by the Event Horizon Telescope, with the black hole’s features more neatly resolved.
Medeiros believes PRIMO could be used to construct images of other mysterious objects. Some of the most interesting processes of planet formation are still invisible to us, even with the best telescopes. Large radio telescopes can record the dust and gas in protoplanetary disks where planets form, and optical telescopes can see fully formed worlds, but the in-between stages of growth don’t come through as clearly. Medeiros thinks systems like PRIMO can improve the resolution of Earth’s most sensitive telescopes, perhaps bringing these mysteries into view.
Despite all the potential, Medeiros says, some scientists are still wary of machine learning. The black box problem remains. (Medeiros notes she built PRIMO from scratch with transparency in mind. But not all astronomical questions can be answered with such bespoke programs.)
ExoMiner is more of a black box, built on existing neural networks and then heavily refined by Valizadegan and his colleagues. But astronomers grew to trust it, especially once it began finding planets.
Valizadegan grew up in Iran, where his love of the night sky began. He often recalls theRubaiyat,a collection of poems by Omar Khayyam, an 11th-century Persian who wrote of the transient nature of life, humanity’s place in the universe, and the forward motion of time. One stanza reads:
For in and out, above, about, below,
’Tis nothing but a Magic Shadow-show,
Play’d in a Box whose Candle is the Sun,
Round which we Phantom Figures come and go.
Valizadegan read these verses as a child and questioned his place in the universe. The idea of other phantom figures out there, dancing around their own candle sun, still keeps him up at night. In the morning, he searches for an answer.
(Earth is a geological oddball in our solar system. This is why.)